Publications
Automatic 2D Cephalometric Landmark Location for Forensic Analysis
Craniofacial Superimposition
Published on May 14, 2025
Written by Guillermo Gómez
Cephalometric landmarks are critical anatomical reference points located on the human face, widely utilized in forensic anthropology for essential tasks such as forensic human identification, facial comparison, anthropometric measurements, and craniofacial superimposition (George, 2007; Stephan et al., 2019; Huete et al., 2015). Identifying and annotating these landmarks traditionally demands considerable expertise, manual effort, and extensive training. This manual process often introduces variability in precision due to factors such as annotator fatigue, experience, and inherent subjectivity (Campomanes-Alvarez et al., 2014).

Addressing these challenges, we have developed an advanced automated method leveraging state-of-the-art deep learning techniques. Our approach utilizes a cascade of convolutional neural networks (CNNs), specifically tailored for the few-shot learning scenarios commonly encountered in forensic contexts, characterized by limited available annotated data. Initially, a pre-trained deformable 3D facial model (3DDFA v2; Guo et al., 2020) approximates the landmark positions. These initial predictions are subsequently refined through a secondary CNN model, trained to precisely correct positioning errors using localized facial image crops around each initial landmark prediction.
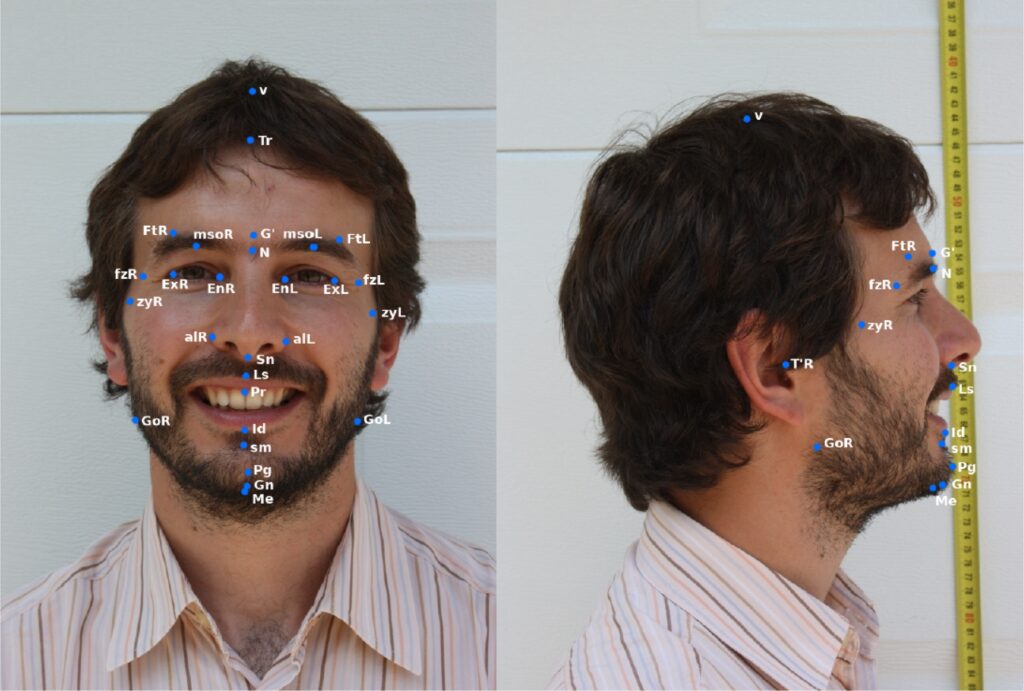
Our proposed cascade method has undergone rigorous evaluation against multiple standard state-of-the-art facial landmark localization approaches. Results demonstrated significant improvements, achieving landmark localization errors two times lower than the best-performing competing method.
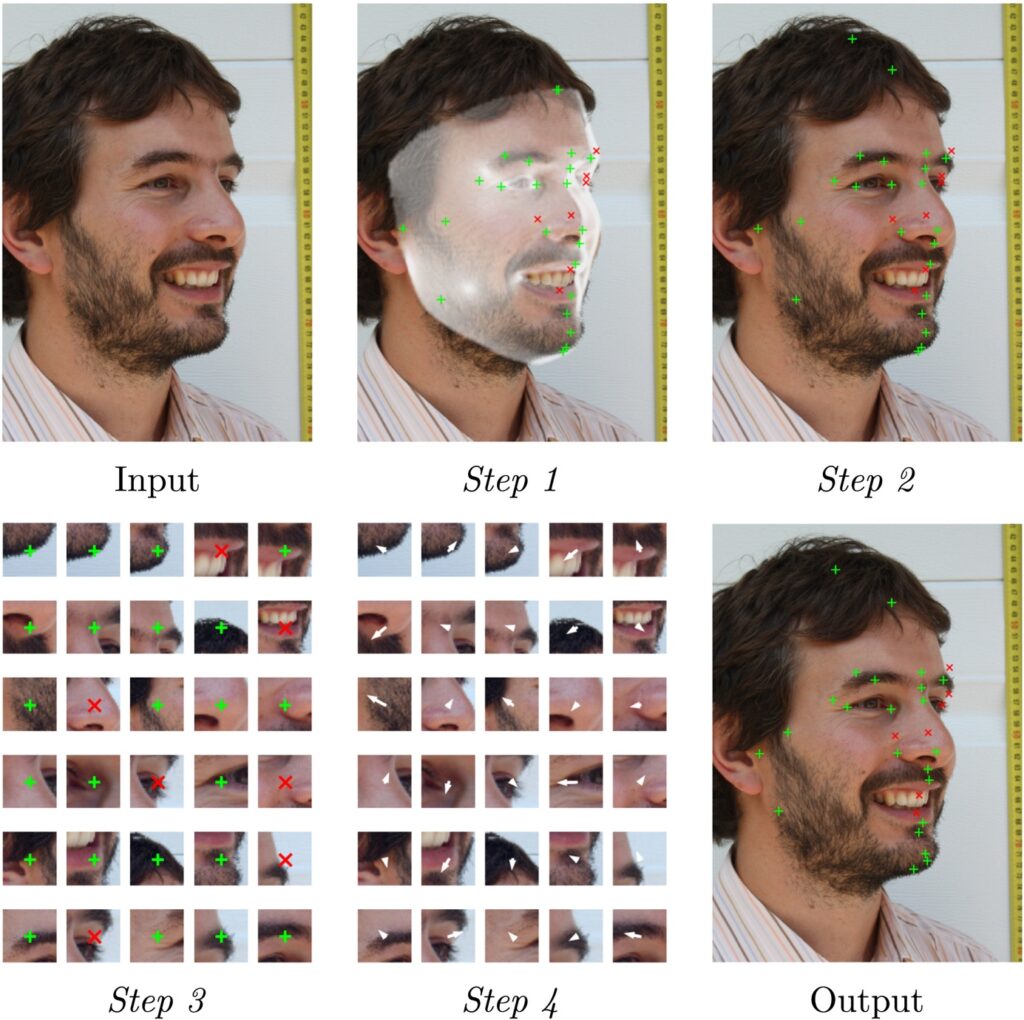
Full process step by step. Notice the different crop sizes depending on the landmark as well as the significant improvement in accuracy.
Further enhancing credibility, we conducted an exhaustive user study involving experienced forensic anthropologists. In this practical evaluation, our automated system’s annotations were compared against those from human experts on real forensic images. The study results were compelling, with expert evaluators considering the automated annotations to be of comparable or superior accuracy in approximately half of the cases tested, thus highlighting the method’s practical utility and reliability for real-world forensic applications.
Considering the outstanding results and proven reliability, this innovative automatic landmark localization method will be integrated into Skeleton-ID, our commercial software solution designed to assist forensic identification using artificial intelligence. Its implementation aims to significantly enhance the speed, accuracy, and reproducibility of cephalometric landmark annotation, effectively eliminating variability linked to annotator fatigue, expertise, or subjective interpretation.
The full publication contains thorough explanations of the methodology, as well as exhaustive cuantitative validation results. The reader can freely access the entire publication through the following link.
References
George, R. M. (2007). Facial geometry: graphic facial analysis for forensic artists. Charles C Thomas Publisher.
Stephan, C. N., Caple, J. M., Guyomarc’h, P., & Claes, P. (2019). An overview of the latest developments in facial imaging. Forensic sciences research, 4(1), 10-28.
Huete, M. I., Ibáñez, O., Wilkinson, C., & Kahana, T. (2015). Past, present, and future of craniofacial superimposition: Literature and international surveys. Legal Medicine, 17(4), 267-278.
Campomanes-Alvarez, B. R., Ibáñez, O., Navarro, F., Alemán, I., Cordón, O., & Damas, S. (2015). Dispersion assessment in the location of facial landmarks on photographs. International journal of legal medicine, 129, 227-236.
Guo, J., Zhu, X., Yang, Y., Yang, F., Lei, Z., Li, S.Z., 2020. Towards Fast, Accurate and Stable 3D Dense Face Alignment. In: Proceedings of the European Conference on Computer Vision (ECCV).
Written by
Guillermo Gómez
Researcher at Panacea Cooperative Research
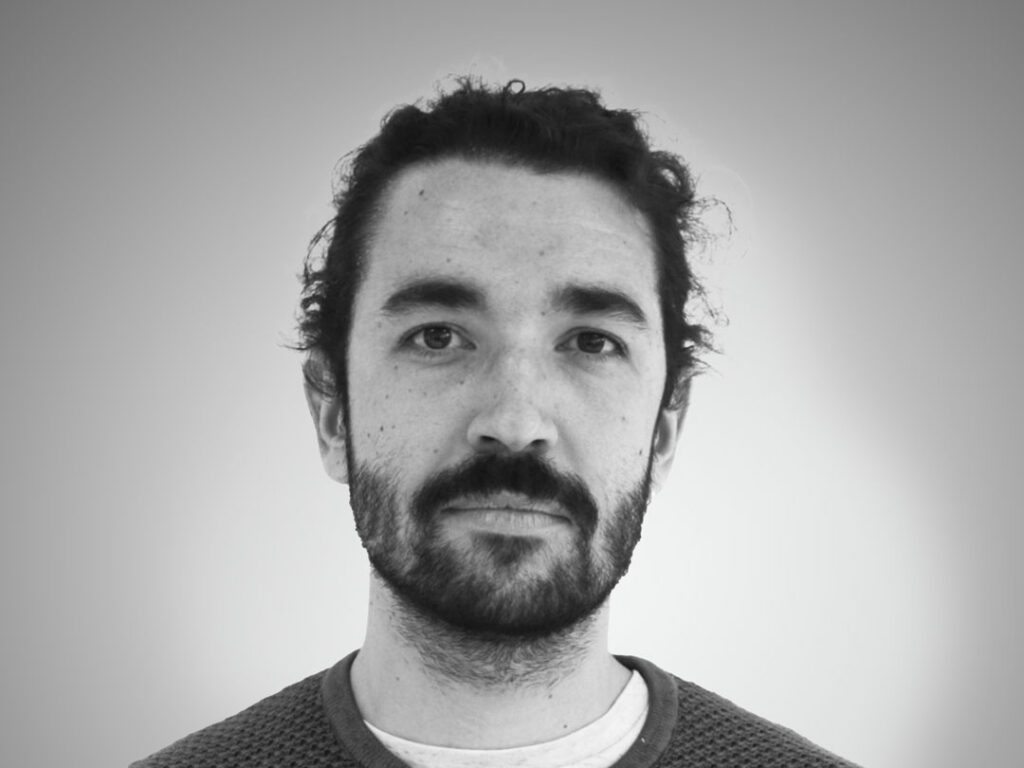